The recent rise of artificial intelligence systems, such as ChatGPT, presents a fundamental challenge for the education sector. The case study we analyze explores the infiltration of artificial intelligence in university examination systems, testing universities' ability to detect the use of AI tools to complete academic assessments. The research was conducted by Peter Scarfe, Kelly Watcham, Alasdair Clarke, and Etienne Roesch at the University of Reading, United Kingdom, and was published in the journal PLOS ONE on June 26, 2024.
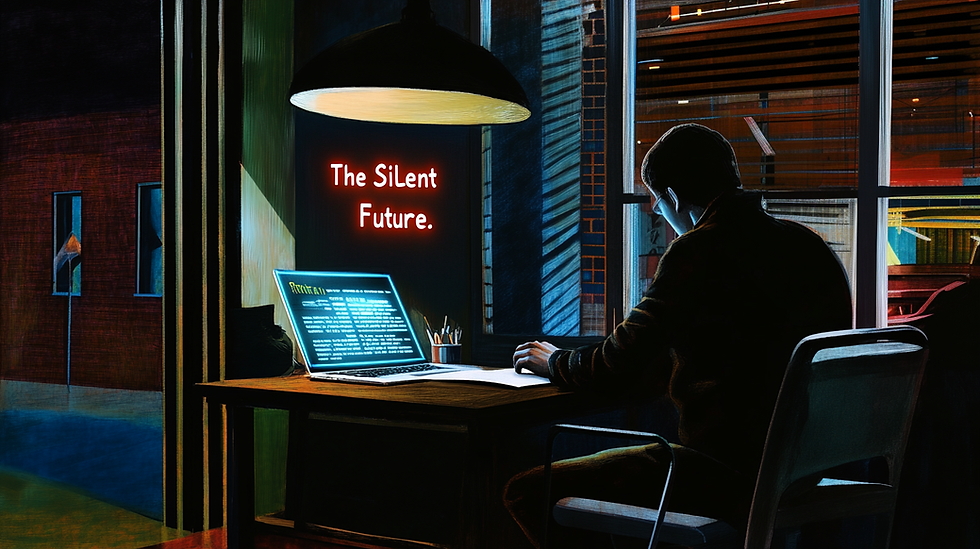
Objective and Methodology
The primary objective of the study was to evaluate the ability of a university to detect exam responses generated by an AI system (specifically GPT-4) and the effect of these responses on the grading system.
The methodology involved injecting AI-generated responses into five modules spread across all years of a BSc Psychology course at the University of Reading. For each module, approximately 5% of the total responses were produced by AI, a manageable number that did not overwhelm the evaluators and ensured uniform coverage.
Two types of exams were used: Short Answer Questions (SAQ) and Essay-Based Questions. The SAQ exams involved answering four out of six questions, within a 2.5-hour time frame. For the Essay exams, students had to respond to a single question with an essay, completed within an 8-hour time limit. All exams were conducted at home, with access to study materials, academic articles, and the internet, which increased the risk of undetected use of AI tools.
To ensure variety in the AI responses, standardized prompts were used for each type of question. However, it was found that the GPT-4 model tended to produce a word count not always in line with the required limit: 160 words for SAQ were often exceeded, while 2000 words for Essays were sometimes insufficient, requiring the use of the prompt "Please continue your answer" to generate more complete texts. In some cases, despite the prompt, the AI generated reference sections that were subsequently removed for consistency with the required response format.
An interesting aspect of the experiment's design was the use of university student aliases created specifically for the submission of AI responses, which allowed for anonymity and ensured that the responses were treated like any other real response by the existing submission and evaluation tools. The AI responses were submitted at the end of the submission period for each exam, faithfully replicating the expected behavior of students.
University Exams and AI: Main Findings
The study's findings highlighted some particularly interesting trends. In addition to the fact that 94% of the AI-generated responses were not detected as suspicious, it was found that AI responses tended to score higher than the average of real students in four of the five modules.
This effect was quantified through various measures, offering deeper insight into the results obtained:
• Grade Distribution: The AI-generated responses showed a concentration in the higher score range. In particular, the grades obtained by AI responses were often placed between the 2:1 and first-class levels. In the grade distribution charts, the AI responses were concentrated in the upper range compared to real student responses, with averages falling within higher classifications.
• Median Analysis: In four of the five modules, the medians of the AI responses were within the 2:1 and first-class range (considered excellent in the British grading system). Only in module P3-M1 did the AI score lower than real students, indicating difficulty in dealing with more advanced and abstract content.
• Score Advantage: In terms of grade advantage, the average margin of the AI responses was about half a classification above the grades of real students, with extreme differences in some cases reaching almost an entire classification level. In module P1-M2, for instance, AI consistently scored in the first-class range, significantly outperforming real students.
• Pass Probability: Through repeated random sampling analysis conducted 100,000 times, it emerged that there was an 83.4% probability that a random sample of AI responses would outperform a random sample of real student responses. In lower-level modules, such as P1-M1 and P1-M2, this probability was nearly 100%. Only in the more advanced module, P3-M1, did AI have a 19% chance of outperforming student responses.
• Pass Rate Analysis: Overall, only 16% of student responses scored higher than the median of AI responses, confirming the superior overall performance of AI-generated answers.
These results suggest that AI not only managed to pass undetected in terms of detectability but also produced significantly higher quality content compared to students in various settings. However, AI showed limitations in tasks requiring more abstract reasoning, as evidenced in module P3-M1.
The Challenge of Detecting AI in Academic Assessments
The analysis of the data collected during the study highlighted a low ability to detect AI-generated responses by the evaluators and the systems currently in use. Only 6% of the responses were flagged for suspicions of academic misconduct, without any explicit reference to the use of artificial intelligence. When the detection criterion was narrowed to cases where AI was specifically mentioned, the detection percentage further dropped to 3%. This highlights a significant difficulty in identifying AI-generated content, despite the efforts of evaluators.
A more detailed analysis revealed that the ability to detect AI was particularly ineffective in Essay-Based questions, where none of the AI-generated responses were identified as suspicious. This could be attributed to GPT-4's ability to generate articulate and coherent responses, which closely resemble the writing style of real students, making it extremely difficult to differentiate between human and AI-generated content. In contrast, for Short Answer Questions (SAQ), where no similarity-checking software was used, some flags were raised. However, even in these cases, the detection rate remained extremely low.
Interestingly, markers (the evaluators assigned to assign grades) flagged only those responses that had particularly anomalous characteristics, such as stylistic quality that was too high for a university exam. In these cases, some markers even attempted to verify the response through AI detection systems, but with poor results. According to the study, most markers lacked sufficient tools and guidelines to identify AI use, and even software designed to detect text generation by language models proved inadequate. For instance, detection systems used, including solutions specifically developed for GPT-4, had a detection success rate of less than 26%, rendering their efforts to counter unauthorized use of AI in assessments practically useless.
These findings suggest a strong need to develop new methods for assessing academic integrity and to enhance markers' training in recognizing signs of AI usage, considering the high probability of false negatives. The analysis of detection rates and obtained grades clearly showed that AI responses were not only practically indistinguishable but also consistently scored better than those of real students, placing themselves in a dangerous position for academic integrity. The adoption of remote proctoring tools and the integration of new pedagogical approaches seem to be the most concrete options for addressing the issue in the future.
Implications for Academic Integrity
This study raises serious concerns about academic integrity and the ability of higher education institutions to deal with the use of AI. With such a high percentage of AI responses going undetected and even managing to achieve higher grades than real students, there is a real risk that students might use AI to gain undeserved academic advantages.
The undetected use of AI raises the risk of a profound distortion of the value of academic qualifications. If AI is systematically outperforming human students and its responses go undetected, there is a danger that grades no longer reflect individual students' competencies, but rather their ability to use technological tools to enhance their performance. This undermines the reliability of the education system as an indicator of merit and acquired knowledge.
In addition, the phenomenon of AI "hallucination," where it produces false but seemingly credible information, adds another layer of complexity. However, the fact that such errors were not evident enough to alert evaluators underscores how difficult it is for teachers to distinguish between authentic and automatically generated responses.
This issue becomes even more critical considering that even the most advanced tools for AI detection have proven ineffective.
Another important aspect is the growing phenomenon of unsupervised exams, a practice accelerated by the COVID-19 pandemic. This exam format offers students a much greater opportunity to use AI tools to complete their assignments. The research showed how home exams, without supervision, are particularly vulnerable to this type of abuse. Since grades assigned to AI-generated assignments were often higher than the average student scores, it is likely that an increasing number of students could be incentivized to use AI to improve their academic performance.
The inclusion of AI technology in academic education may be inevitable, but it is necessary for clear norms to be established on how and when it is permissible to use it. A possible response could be to revise assessment methods, integrating approaches that are more challenging to tackle using AI. For example, practical assignments, oral assessments, or supervised group projects could reduce the impact of unauthorized use of technological tools. Additionally, it might be helpful to teach students how to use AI ethically and responsibly, preparing a generation of graduates who can leverage these technologies without resorting to academic misconduct.
Conclusions
The integration of artificial intelligence in university examination systems represents a crucial turning point for the education sector, highlighting deep vulnerabilities in traditional assessment methods and raising fundamental questions about academic integrity and the future of education. The analyzed study reveals an uncomfortable reality: AI is not only difficult to detect but often outperforms student responses, demonstrating that current evaluation criteria may favor content generated by algorithms rather than by human understanding. This underscores a paradox: academic success may depend less on individual capability and more on technological competence, undermining the meritocratic principle underlying higher education.
University exams and AI open up scenarios of great strategic relevance, characterized by considerable complexity and a wide range of implications. First, a systemic challenge emerges: if AI can produce undetectable and high-quality responses, this forces institutions to reconsider not only detection methods but also the very concept of competence assessment. The ability to memorize information or write a well-structured essay may no longer be the benchmark for measuring learning. It becomes essential to redefine educational goals, focusing on skills that AI cannot easily replicate, such as critical thinking, creativity, and the ability to integrate interdisciplinary knowledge.
This shift requires a transition from a reactive evaluation model to a proactive one. Universities must develop approaches that not only detect AI usage but consider AI itself as a teaching tool to be ethically integrated. For example, rather than banning the use of AI, students could be evaluated on their ability to collaborate with it effectively, transparently, and innovatively. Such an approach would not only reduce the risk of abuse but also prepare students for a job market where AI is increasingly pervasive.
Another crucial element is the urgency of creating a resilient educational ecosystem. The pandemic has accelerated the adoption of unsupervised exams, but this format has proven particularly vulnerable to AI abuse. Institutions must balance the need for flexibility with the requirement to ensure the integrity of results. Solutions like remote proctoring, while useful, risk compromising trust between students and universities if perceived as intrusive. Therefore, it is essential to develop less intrusive but more effective technological tools and invest in a culture of transparency and ethics.
Finally, the implications extend beyond education, affecting the job market and society at large. If academic qualifications lose credibility, there is a risk of a crisis of trust in academic institutions, with direct consequences on the employability of graduates. Companies, for their part, will need to adapt their selection processes to distinguish between real skills and abilities derived from AI usage. This requires greater collaboration between universities and employers to define assessment standards that reflect candidates' real capabilities.
In summary, the advent of AI in university examination systems represents not only a technological challenge but also a unique opportunity to rethink education and its role in society. The future of learning will not be determined by the ability to avoid AI but by the ability to coexist with it, leveraging it as a catalyst for more authentic, inclusive, and real-world-oriented education.
Comments