Artificial intelligence (AI) is rapidly transforming the field of scientific research, making processes faster and providing more accurate insights compared to traditional methods. The research by Eberle et al. (2024) is a significant example of how AI can be used to analyze large volumes of historical data, providing insights that would have been difficult to obtain with conventional techniques. The study examined a large corpus of historical texts, using advanced machine learning techniques to analyze and compare versions of various astronomy and mathematics texts, with a particular focus on the spread of mathematical knowledge between 1500 and 1650. Eberle et al. applied a process of atomization of the texts, breaking them down into individual units of data such as tables and numbers, and then recomposing them to identify patterns and trends in scientific knowledge. The results showed a significant reduction in the entropy of printed content between 1540 and 1560, indicating a growing homogenization of knowledge, often influenced by figures like Oronce Finé, whose work was widely republished. In this article, we will explore the application of AI in historical research, focusing on this specific case of the spread of mathematical knowledge in 16th-century Europe, using significant data from the study.
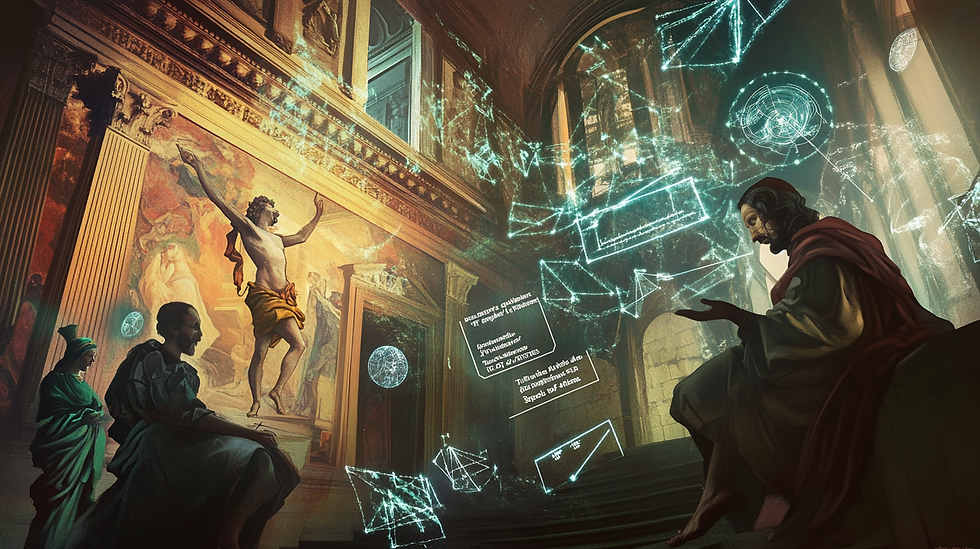
Atomization and Recomposition: An AI-Based Approach for Historical Analysis
The study by Eberle et al. is based on an innovative process called "atomization and recomposition" to analyze a large corpus of historical texts in astronomy and mathematics, known as the Sacrobosco Collection. The main goal is to make complex historical data more accessible and usable, thus enabling the identification of significant patterns and trends that would otherwise remain hidden.
The process of atomization begins with the digitization of original works, a fundamental step that transforms printed texts into a machine-readable form. These digital works are then broken down into smaller units of data: words, phrases, tables, and numbers. Each element is labeled and classified so that the ML model can subsequently analyze it accurately. The convolutional neural network (CNN) used for the analysis was trained to identify specific elements such as numerical tables and diagrams, which are particularly useful for studying scientific content.
The recomposition approach, on the other hand, involves reintegrating the atomized data to identify hidden relationships and understand how scientific knowledge evolved over time. For example, thanks to the recomposition of data, researchers were able to compare different editions of the same text, analyzing which elements were added, modified, or removed. This allowed a better understanding of how mathematical ideas spread and were modified in response to cultural, political, and economic factors.
The use of machine learning allowed the calculation of entropy in publications between 1540 and 1560, highlighting a significant reduction in this parameter. This decline suggests a trend towards the homogenization of knowledge, presumably to meet the needs of an increasingly standardized academic audience.
Moreover, the atomization and recomposition approach enabled the identification of anomalies, such as the coexistence of old and new theories within the same historical context. One example of this was found in the tables of climatic zones, where the ancient conception of climatic zones in seven parts continued to coexist with the more modern division into 24 zones. This type of discovery helps researchers better understand how new knowledge does not always replace existing ideas but often develops in parallel, influencing each other.
The entire process of atomization and recomposition was made possible by integrating advanced artificial intelligence models and natural language processing (NLP) techniques. The use of NLP was fundamental for the semantic analysis of texts, allowing the model to distinguish between different types of information and understand the context in which certain scientific terms were used. This made it possible to identify changes in the meaning and use of scientific terminology over time.
Overall, the atomization and recomposition approach not only accelerates the research process but also offers new perspectives for analysis. It allows researchers to analyze historical texts quantitatively, identifying patterns and trends on a large scale, and conduct more detailed qualitative analyses on specific elements. This type of integration between AI and historical research represents a significant step forward in understanding the evolution of human knowledge.
Significant Data: Diffusion and Homogenization of Knowledge
The data collected from the study show how the spread of mathematical knowledge between 1500 and 1650 was characterized by phases of intense publication and republication, often driven by market mechanisms that encouraged the production of new editions in quick succession. This phenomenon facilitated the rapid dissemination of scientific knowledge but also led to greater standardization of content.
Entropy, a measure of content variability, showed a decrease, suggesting that the same information was repeated in multiple publications, thereby reducing the diversity of available knowledge. This reduction in entropy was largely attributed to the influence of Oronce Finé, a French royal mathematician, whose works were continually republished during this period. The repeated reprints of his works helped disseminate a standardized view of mathematics, which had a lasting impact on the scientific formation of the time.
Another relevant aspect that emerged from the study is the assessment of the geographical distribution of knowledge in terms of entropy of publications. Cities with lower entropy scores, such as Wittenberg and Frankfurt am Main, showed highly homogeneous production, presumably due to political and religious control over scientific education. In these cities, the standardization of content was encouraged by academic institutions that aimed to consolidate a unified vision of science. This type of control led to a reduction in the diversity of available content, contributing to the formation of shared and consolidated knowledge.
In contrast, places with greater diversity in editorial production, such as Venice and Paris, favored a wider variety of scientific content. These cities were major cultural and commercial centers, with greater editorial freedom and a higher demand for diverse works. This fostered the publication of innovative texts and the introduction of new ideas, helping to maintain a certain heterogeneity in the diffusion of knowledge. The variety of publications in these centers allowed for the coexistence of different approaches to mathematics and facilitated the development of new theories, in contrast to the standardization trend observed in other regions.
Another significant finding concerns the coexistence of old and new theories within the same publications. This phenomenon highlights how the spread of knowledge was not a linear process but rather a complex interaction between tradition and innovation.
This dynamic coexistence of old and new had important implications for the scientific and cultural formation of 16th-century Europe. It allowed for a gradual transition to new ideas while maintaining continuity with the past. This balance between continuity and innovation was fundamental for scientific progress, as it allowed new ideas to take root without creating a drastic break with established knowledge.
Implications for Historical Research
The AI-based approach has allowed not only the tracing of the diffusion of knowledge but also the identification of anomalies and deviations from dominant trends.
Furthermore, the use of AI for the analysis of historical data makes it possible to examine the processes of homogenization and diversification of knowledge in greater detail than in the past. The ability of AI to identify patterns and relationships on a large scale enables researchers to better understand the mechanisms through which knowledge spreads and stabilizes. This is particularly important for understanding how academic and political institutions have influenced the standardization of knowledge and how market dynamics have contributed to the reproduction and dissemination of scientific content.
The implications of this approach for historical research go beyond the simple understanding of the spread of mathematical knowledge. The integration of machine learning techniques allows researchers to address broader questions, such as the role of academic communication networks, the impact of social and economic dynamics on scientific production, and the resilience of scientific theories in the face of cultural and political changes. This type of analysis opens new perspectives for historical research, allowing researchers to explore complex phenomena and develop new hypotheses based on empirical data.
Another important aspect concerns the possibility of using AI to identify the influence of individual actors or institutions in the diffusion of knowledge. The study by Eberle et al. highlighted how the republication of Oronce Finé's works played a fundamental role in the standardization of mathematical knowledge of the time. This type of analysis can be extended to identify other individuals or institutions that had a significant impact on the formation of scientific knowledge, thus providing a more complete understanding of the historical dynamics of knowledge production.
Finally, the use of AI in historical research also raises interesting methodological questions. The integration of advanced technological tools requires a critical reflection on the limitations and potential of these tools. It is important for researchers to play an active role in interpreting the results produced by machine learning models, ensuring that analyses are adequately contextualized and that conclusions are based on a thorough understanding of historical data. AI can therefore be seen as a complementary tool that amplifies the research capabilities of historians without replacing their critical and interpretive role.
Conclusions
The use of artificial intelligence in the historical analysis of the spread of knowledge, as exemplified by the study by Eberle et al., opens up strategic perspectives for companies operating in the field of knowledge and technology. The process of "atomization and recomposition" represents an innovative methodology that allows the breakdown and reassembly of large amounts of data to obtain insights that are not detectable through traditional human analysis. This model makes it possible to create patterns of meaning that identify evolutionary trajectories and changes in trends, highlighting how the adoption of certain knowledge can spread and take root based on interactions between cultural, economic, and social factors.
For companies, the application of this type of AI in historical data analysis suggests an approach to big data that goes beyond quantitative processing to understand the evolutionary history of consumer or market behavior. The ability to identify moments of "reduction in entropy," or standardization of information, not only provides a picture of knowledge stabilization but also offers a valuable indicator for evaluating periods when a certain level of knowledge consolidates and becomes an industry standard. Similarly, moments of greater "informational variance" can identify periods of diversification or innovation that can guide companies in developing products or services at a strategic time.
The coexistence of old and new knowledge highlighted by the study, and the tendency towards integration rather than outright replacement, offers a fundamental lesson for business knowledge management. Companies should consider that innovations do not always need to replace established methodologies but can instead create a useful synergy if implemented in parallel, respecting the absorption times and familiarity of their market or employees. This coexistence suggests that gradual integration of new technologies is not only prudent but can be essential to ensure acceptance and effectiveness.
Finally, the use of AI as a "complementary tool" to human interpretation raises a crucial point for organizations implementing advanced AI: the critical value of human interpretation. AI offers enormous capabilities for analysis and automation, but the resulting data require human expertise to translate the identified patterns into strategic decisions. This means that companies should structure their teams to integrate AI experts and data analysts with profiles capable of providing context, insights, and historical experience of business phenomena, avoiding the risk that statistical and ML models are misunderstood or used non-strategically. In summary, AI becomes a tool for the conscious evolution of knowledge, a resource to improve business decision-making processes, and a means to facilitate continuous adaptation in a dynamic market context.
Comments