In contemporary business, artificial intelligence (AI) is increasingly woven into critical processes, from data-driven forecasting to algorithmic decision-making. The need for responsible governance of these intelligent systems is no longer a tangential concern; it stands at the core of organizational leadership. A recent research effort titled “Responsible artificial intelligence governance: A review and research framework,” authored by Emmanouil Papagiannidis, Patrick Mikalef, and Kieran Conboy, in partnership with institutions such as the Norwegian University of Science and Technology and the National University of Ireland, underscores how companies can benefit from adopting ethical principles in AI. By doing so, they mitigate potential reputational, financial, and societal risks, ensuring that the technology remains a force for constructive growth rather than a catalyst for harm.
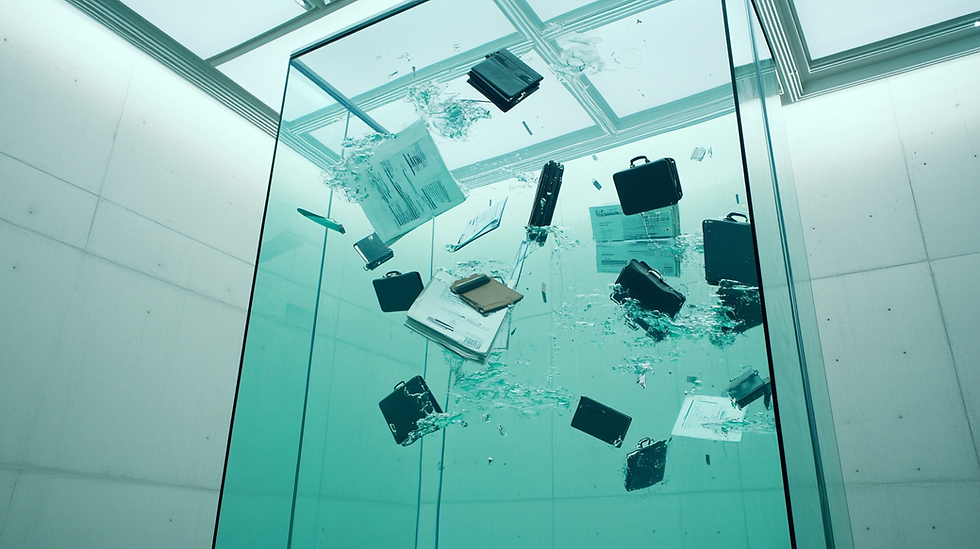
Understanding Responsible AI Governance: Key Concepts and Definitions
Organizations across diverse sectors have embraced AI, but the need for responsible AI governance remains critical in managing ethical challenges posed by these systems. The foundational idea behind AI, according to this research, involves a system’s ability to recognize patterns, interpret large datasets, draw inferences, and learn continuously to advance various organizational and social objectives. Yet alongside this remarkable potential for predictive analytics and streamlined processes, there arise challenges such as unintended discrimination, lack of clarity in algorithmic decisions, and ongoing debates over accountability when machines make high-stakes determinations.
Through a thorough screening of academic literature—starting with over a thousand articles and narrowing it down to a carefully selected set of highly relevant papers—this study reveals a pronounced fragmentation in how scholars and practitioners address responsible AI governance. While principles like fairness, accountability, transparency, and privacy have been articulated, there is no universal framework tying these ideas together into operational realities. One emblematic case involved Amazon, where an automated candidate-screening tool ended up disadvantaging qualified female applicants. That scenario underscored the dire need for more concrete guidelines on how to integrate ethical considerations into each step of AI’s lifecycle, from the earliest design decisions through ongoing performance monitoring.
The research posits that responsible AI governance must be anchored in well-defined standards that strive to prevent detrimental outcomes for individuals and society at large. This encompasses attention to diversity, equitable treatment, technical robustness, explainability, and overall social welfare. Importantly, the investigators note that building a cohesive governance structure requires alignment with existing corporate values and responsiveness to evolving regulatory environments. Everyone involved—ranging from AI developers and data scientists to managers, external auditors, and end users—ought to be empowered with the necessary skills and information to uphold ethical imperatives, such as evaluating algorithms deemed “black boxes,” setting up rigorous auditing procedures, and engaging in continuous training.
Structural Decisions for Effective AI Governance
A central insight from the study is that structural decisions within an organization have a profound effect on whether responsible AI governance will genuinely take root. Many companies have begun creating oversight committees tasked with reviewing and guiding AI initiatives. Such bodies might clarify responsibilities around approving new algorithmic features, ensuring the quality of input data, or deciding when and how to intervene if things go awry. By distributing accountability throughout the entire organizational hierarchy, leaders are better positioned to recognize potential risks early and respond swiftly.
Adopting clear protocols that connect executives, software developers, risk managers, data scientists, legal advisors, and even marketing teams can help cultivate a culture where ethical concerns are not afterthoughts but integral components of AI deployment. On a vertical level, these structures confirm that each rank—from mid-level managers to boards of directors—knows its role in overseeing systems that might inadvertently discriminate or breach privacy. Horizontally, different departments can collaborate to incorporate user feedback, adhere to relevant regulations, and safeguard a company’s brand reputation.
Another vital consideration is the external environment. Businesses today rarely function in silos; AI solutions often draw upon outside data from suppliers or sector-wide ecosystems. Hence, a comprehensive responsible AI governance model may require establishing security contracts with third parties or adopting cross-organizational frameworks ensuring data integrity, confidentiality, and compliance with national and international laws.
Practical Frameworks in Responsible AI Implementation
Beyond organizational charts and committees, the study emphasizes the importance of practical procedures for turning abstract ethical guidelines into daily routines. A key focal point is data management: building AI on representative, up-to-date, and unbiased datasets is critical to reduce the risk of producing skewed or prejudiced outcomes. Similarly, robust privacy policies and traceable mechanisms for accessing data can bolster compliance with regulations like the GDPR in the European Union.
Another cornerstone is continuous testing and monitoring. Algorithms should undergo rigorous evaluations not just at deployment but periodically throughout their operational life. This involves measuring accuracy, reliability, and any drift in performance. Researchers have advocated for a retrospective review framework in cases where AI-based decisions lead to erroneous or harmful results. By analyzing the circumstances that triggered such missteps—sometimes called “retrospective disaster analysis”—organizations can refine or retrain their models and improve detection of anomalies.
Moreover, building fallback protocols into the system design allows a rapid response to attacks like “data poisoning,” where adversarial inputs corrupt the learning process. Technical robustness remains an ongoing mandate, requiring collaboration between security experts and AI developers to ensure that vulnerabilities are addressed early and systematically. With the rise of generative AI tools such as ChatGPT, new ethical dilemmas also emerge around content misuse and the extent to which automated models can overshadow human judgment. The study notes that well-crafted governance mechanisms help mitigate power imbalances between those who build AI systems and those who utilize—or are affected by—them.
Human-Centric Approaches to Responsible AI Governance
A recurring theme in the research is that even the most detailed policies can falter if the human dimension is neglected. Collaboration, open communication, and stakeholder involvement often separate successful AI governance efforts from superficial ones. Bringing together voices from legal counsel, domain experts, consumer advocates, and vulnerable communities early in the planning phase can expose hidden assumptions embedded in AI systems, such as biases in historical data or oversights in how users might interact with new applications.
Engaging diverse stakeholder perspectives also builds trust: if a machine-learning model determines, for example, an individual’s eligibility for a loan, those affected by the system need clear and comprehensible explanations. Transparent communication, in turn, not only addresses the black-box anxiety but also enhances brand perception. The research underscores how timely efforts in upskilling employees—whether they be software engineers, analysts, or managers—lay the groundwork for responsibly adapting to AI-driven transformations. Team members who understand both the capabilities and the limitations of AI are more likely to flag ethical pitfalls before they escalate.
This human element includes helping employees, managers, and leaders develop emotional intelligence around AI. When staff feel threatened by automation or fear a major shift in responsibilities, it can lead to obstructive behavior or silent non-cooperation. The researchers note that training, simulations, and real-world case studies all help organizations dispel misinformation and build consensus on principled AI usage. In a broader sense, a well-educated workforce becomes a competitive advantage, as they can innovate in ways that strike a balance between ethical concerns and market demands.
Ethical AI: Future Trends and Challenges
The study draws attention to how ethical AI practices can shape the long-term value proposition for a company. As markets tighten and public scrutiny increases, an enterprise that demonstrates robust data safeguards and a genuine commitment to fairness can draw in ethically conscious investors, partners, and clients. Such alignment with Environmental, Social, and Governance (ESG) ideals can reduce legal risks, avert negative media coverage, and strengthen the confidence of regulators.
Additionally, transparent AI governance fosters healthier internal cultures, mitigating fears that new technologies will eradicate human involvement or erode professional dignity. Clear protocols ensuring human oversight and real accountability for automated decisions often lead to higher employee retention and a sense of shared purpose. Meanwhile, the public’s growing familiarity with AI’s capabilities and limitations informs corporate strategies. The study suggests that the influx of public opinion on AI’s impact—particularly regarding generative systems or advanced machine learning—gradually reshapes corporate norms and influences the direction of policy.
Looking forward, the authors anticipate further ethical dilemmas concerning ownership, authorship of AI-generated content, and the potential displacement of skilled workers. Organizations that invest time and resources to address these emerging questions early are more likely to thrive under shifting regulatory conditions. By embedding robust responsible AI governance protocols into strategic planning, organizations can mitigate unintended outcomes, harness technology for constructive ends, and cultivate resilience in an evolving landscape.
Responsible AI Governance: Conclusive Insights
Ultimately, the research underscores that embracing responsible AI governance is not just a matter of compliance but a pathway to sustainable success in a digitized era. Though AI is often viewed through the lens of technical prowess, the study reminds us that it is equally a cultural, ethical, and managerial undertaking. Leaders must synergize technical audits, transparency methods like explainability tools, and a culture of proactive questioning. Lacking these elements, piecemeal governance efforts may fall short—particularly given how swiftly AI can adapt and influence real-world conditions.
A notable realization is that many of the problems posed by AI mirror longstanding issues in software development and data quality assurance, though on a grander scale. By adopting a systemic mindset—one that acknowledges AI’s capacity to evolve continuously—businesses can strengthen their responsible AI governance by incorporating dynamic learning into their processes. In doing so, they empower teams to identify possible harm before it becomes irreversible, while simultaneously nurturing new digital services that reflect both consumer priorities and moral imperatives.
Rather than viewing responsible AI governance as an abstract or ceremonial gesture, the research reveals its tangible benefits in shaping trust, cooperation, and innovation within the broader socio-economic environment. For enterprises aiming to remain competitive, striking this balance between technological ambition and accountability has become an essential ingredient for long-term relevance.
Comments