Optimizing Teaching with POSR: Intelligent Segmentation and Retrieval
- Andrea Viliotti
- 19 nov 2024
- Tempo di lettura: 11 min
Educational conversations, such as tutoring sessions, are often complex and multifaceted, especially when they revolve around reference materials like exercise sheets. Segmenting these discussions and linking them to appropriate references is a significant challenge in improving teaching quality. The Problem-Oriented Segmentation and Retrieval (POSR) framework, developed by a team of researchers from Stanford University comprising Rose E. Wang, Pawan Wirawarn, Kenny Lam, Omar Khattab, and Dorottya Demszky, represents an integrated approach to addressing segmentation and retrieval of relevant information in conversations, aiming to enhance the organization and effectiveness of the teaching process.
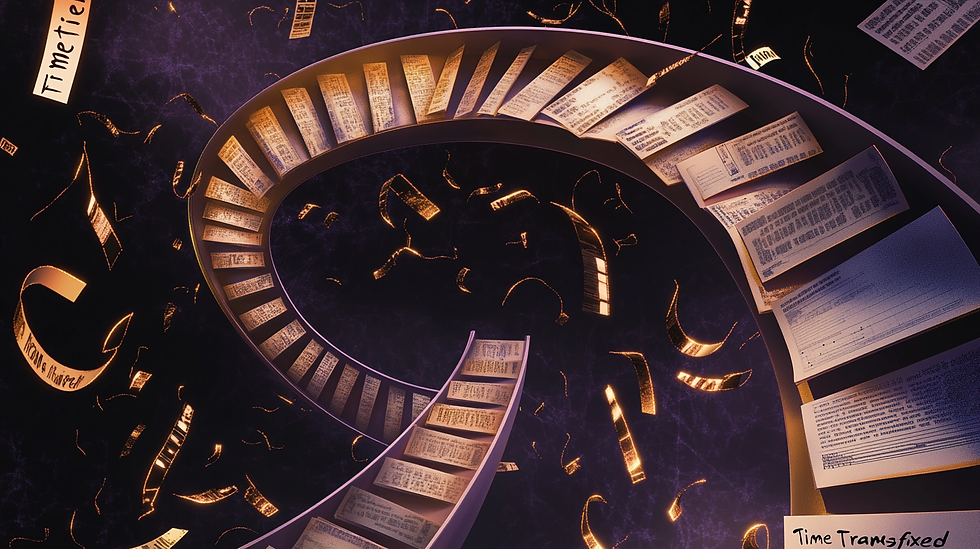
What is POSR?
POSR is a tool that combines two essential functions in the field of natural language processing: discourse segmentation and information retrieval. These two processes, typically considered separately, are integrated into POSR to provide a more complete and structured view of conversations, such as those occurring during a lesson or an educational session.
Discourse segmentation involves breaking down a conversation into smaller, meaningful parts. For example, in a school lesson, the discourse can be divided into moments such as explaining a new concept, discussing an exercise, or a question-and-answer session between tutor and students. This subdivision helps better organize the content and identify key moments in the dialogue. Information retrieval, on the other hand, focuses on associating each of these parts with specific resources, such as notes, exercises, or key concepts. For example, if a mathematical rule is explained during a lesson, POSR automatically links that segment to the relevant documentation or related problems.
An innovative aspect of POSR is the use of contextual information to make these operations more accurate. Traditionally, segmentation methods follow rigid rules, such as recognizing pauses or specific linguistic patterns, but POSR goes further. For example, it can adapt to how a tutor answers students' questions, understanding if a response represents a deeper exploration of an already explained concept or the introduction of a new topic. This contextual approach allows creating segments that better reflect the real flow of the lesson, making it easier to understand exactly when a concept was addressed.
From a technological perspective, POSR uses a mixed approach, combining traditional methods like TextTiling (a technique that divides texts based on topic changes) with advanced language models like GPT-4 and Claude. These more recent models, capable of analyzing large amounts of text, can capture the nuances of human language, such as changes in tone or implicit references. For example, if a tutor uses a metaphor to explain a scientific concept, POSR can recognize this metaphor as part of the educational segment, whereas a traditional method might ignore it.
To evaluate the quality of POSR's work, specific metrics have been introduced. One of these is the Segmentation and Retrieval Score (SRS), which takes into account both the accuracy with which discourses are divided and the correctness with which the segments are linked to reference materials. For instance, this metric checks if a mathematical explanation is associated with the correct practical exercises. Additionally, metrics like Time-Pk and Time-WindowDiff also evaluate the duration of segments, which is particularly useful in educational contexts, where the time dedicated to each topic is crucial. For example, these metrics can indicate if the time spent explaining a concept is proportional to its complexity.
An additional feature of POSR is its ability to analyze how time is used during a lesson. This means, for example, that it is possible to see how much time a tutor spends explaining a complex concept compared to a simpler topic. These analyses help improve the quality of teaching by offering practical suggestions on how to better distribute time and resources. If a tutor spends too much time on one part of the lesson, POSR can highlight this aspect and propose strategies to better balance explanations, fostering more effective learning for students.
In summary, POSR represents an advanced system that not only organizes and links information intelligently but also provides useful tools to improve teaching through the analysis of time and the language used by tutors. This makes it particularly useful for those seeking to optimize lessons and personalize them according to students' needs.
Application of POSR in the Educational Context
The application of POSR, a system for analyzing educational conversations, in the educational context proves particularly useful for identifying critical points within interactions between tutors and students. An interesting aspect emerging from linguistic studies is that tutors who spend more time explaining a problem tend to use a more conceptual approach, focusing on the principles and fundamental ideas. On the contrary, tutors who spend less time prefer procedural explanations, concentrating on the operational steps to solve the problem. This knowledge can significantly improve teaching quality, as it helps identify the best strategies to foster deeper and more lasting learning.
A practical example is the analysis of a math lesson. If a tutor spends extra time explaining why a particular formula works, students tend to understand the concept better and remember it longer. Conversely, quick explanations that only illustrate the necessary steps often lead to superficial learning, useful only in the short term.
POSR is also helpful in assisting tutors in dynamically adapting their lessons. During a lesson, it is common for the pace and depth of explanation to need modification based on student responses or encountered difficulties. Thanks to POSR, it is possible to monitor in real-time how explanations are structured and how time is managed. For example, if the system detects that a concept was covered too quickly compared to students' comprehension standards, the tutor can be advised to slow down and provide further detailed explanations. In a concrete case, during a geometry lesson, if POSR detects that many students are not understanding the Pythagorean theorem, the tutor can be encouraged to provide practical examples, such as calculating the diagonal length of a room, to clarify the concept.
Another area where POSR proves valuable is in designing educational programs. Through the analysis of recorded conversations, it is possible to identify concepts that require more attention because they are challenging to learn. For example, in a dataset like LessonLink, which collects over 3,500 lesson segments and more than 24,300 minutes of teaching related to 116 SAT® math problems, one can observe that problems requiring probability calculations are often more difficult for students than other topics. With this information, curriculum developers can include more targeted exercises or additional materials to better address these complex concepts.
An additional advantage of POSR concerns tutor training. By analyzing lessons from expert tutors, it is possible to create models of best practices that can be shared with less experienced tutors. This process allows standardizing teaching methodologies, improving the overall quality of lessons. For example, a novice tutor can learn from a model that a successful explanation often includes a balanced mix of visual elements, such as charts or drawings, and verbal explanations that connect the new concept to students' existing knowledge.
LessonLink is a dataset designed to support the application of POSR, configuring itself as a valuable resource for the educational sector. This tool collects detailed information on how tutors manage time and structure explanations during a lesson. In addition to offering insights for improving teaching practices, it provides practical examples on how to address complex concepts in concrete contexts. For instance, by analyzing a segment dedicated to explaining the concept of a derivative, it is possible to identify the most effective strategies, such as using intuitive explanations based on analyzing curve slopes or more rigorous approaches grounded in technical definitions.
These applications of POSR demonstrate how a system based on conversation analysis can have a concrete and measurable impact on the quality of education, providing tools to make learning more effective and tailored to students' needs.
Why POSR is Important
Among POSR's key features is its ability to segment lessons intelligently, identifying points requiring greater attention and providing targeted support to teachers. This approach allows concentrating explanations on the most complex segments, adapting depth to students' preparation levels, and ensuring more effective learning.
Another advantage of POSR lies in optimizing teaching time, a crucial element for educators. Time management is often a complex challenge, as an unbalanced use can lead to incomplete content coverage or superficial understanding. Through analyzing the temporal dynamics of lessons, POSR identifies areas where too much or too little time is invested and suggests solutions for a more effective balance. This ensures uniform content coverage, allowing all students to acquire essential knowledge.
POSR is particularly useful in individual tutoring, where personalization is essential. Since tutoring sessions require quick and targeted responses, the system helps tutors quickly identify students' difficulties and intervene with clear and specific explanations. POSR's ability to provide immediate feedback facilitates timely interventions, significantly improving the effectiveness of educational support.
In educational contexts with multiple tutors, POSR helps ensure consistency in teaching. The variability in methods used by different educators can affect learning quality. POSR allows defining common guidelines through segmentation and information retrieval, improving lesson consistency. Additionally, the analysis of collected data helps identify the most effective teaching practices, providing valuable support for continuous tutor training and improvement.
The integration of POSR into digital learning platforms represents another significant aspect. Thanks to its ability to process large amounts of data, POSR supports the development of e-learning systems that can automatically adapt to students' needs. This approach makes large-scale personalized learning possible, improving both the accessibility and effectiveness of education.
A distinctive element of POSR is its ability to provide a quantitative assessment of educational impact through specific metrics such as the Segmentation and Retrieval Score (SRS). This analytical tool allows precisely measuring the effectiveness of adopted methodologies, supporting data-driven decisions to optimize teaching practices. Studies have shown that treating segmentation and information retrieval as a single process, rather than separate activities, significantly improves results. In particular, there was a 76% increase in joint metrics and a 78% increase in segmentation metrics compared to traditional approaches. Furthermore, integrating these functions into a single system reduces costs and increases overall efficiency.
Despite its many advantages, POSR has some limitations related to the high costs of large language models, especially for processing long texts. This represents an obstacle to scalability in educational contexts with limited resources. To address this issue, one future challenge will be developing more accessible and efficient open-source solutions that maintain the same quality levels.
The LessonLink Dataset: A Resource for Education
The LessonLink dataset is one of the first resources to systematically integrate tutoring conversations with specific problems, creating a detailed connection between each lesson segment and the topics covered. This dataset was created in collaboration with Schoolhouse.world, a peer-to-peer tutoring platform supporting over 80,000 students globally with the help of approximately 10,000 volunteer tutors. LessonLink's main focus is on SAT® math problems, a standardized test used for college admissions in the United States.
One of LessonLink's distinctive features is the granularity of the annotations. Each lesson has been segmented into distinct educational units, such as introductions, exercises, and reviews of specific problems from the worksheet. This detailed segmentation, which also relies on precise start and end timestamps for each segment, offers an in-depth analysis of how tutors manage time and respond to students' various needs. This allows identifying moments when a particular concept requires more attention and at which stages of the lesson the teaching strategy needs adaptation.
The LessonLink dataset was designed to be an open-source resource, available to the educational research community to promote innovation in teaching and tutoring. Data collection was conducted rigorously, ensuring participants' de-identification to preserve their privacy, using a specialized library called Edu-ConvoKit.
LessonLink, with its unique features and rich data, has the potential to significantly influence the design of educational programs and teaching practices.
Dataset LessonLink: Tools and Opportunities for the Future
The availability of such a rich and structured dataset represents an essential resource not only for the analysis of educational conversations but also for developing artificial intelligence tools applied to education. Machine learning models can be trained on LessonLink to improve automatic segmentation and information retrieval in other teaching contexts, making POSR technology replicable and adaptable to a wide range of educational scenarios. This aspect is crucial to making personalized education accessible on a large scale, especially in a world where distance learning and digital technologies are becoming increasingly prevalent.
Conclusions
The POSR framework introduces a new perspective into the educational context, but its real potential emerges only when viewed through a strategic lens that goes beyond the technical boundaries of segmentation and retrieval. The true innovation of POSR does not lie solely in its ability to optimize lessons but in the possibility of redefining the relationship between knowledge and time—a central and often overlooked theme in designing educational and training systems.
POSR highlights a fundamental principle: time is a pedagogical resource, not just an operational constraint. This vision implies that how time is used, distributed, and adapted within lessons can profoundly transform learning quality. It is not just about doing more in less time but about recognizing which moments require more expansion to foster deep learning and which can be simplified without losing value. This ability to read and intervene on the "times of knowledge" can be translated into any training field, corporate or academic, as a strategic metric to improve the quality of interactions and outcomes.
But there is another subtler and less explored aspect. POSR, in its process of segmentation and contextual linking, brings to light the "geography of learning." Each segment is not just a block of content but a space of interaction, a junction where language, thought, and attention converge. Contextual information retrieval is not just about improving accuracy; it draws an invisible map of the cognitive and communicative dynamics that develop between tutor and student. This map is a powerful tool because it makes visible what usually remains implicit in educational practices: critical points, nodes of complexity, areas of opportunity to expand understanding or intervene in motivation. It is an epistemological evolution because it allows seeing learning not as a straight line but as a stratified ecosystem of interactions.
Another crucial point is the concept of "latent feedback." With POSR, the tutor not only receives direct indications on where and how to improve but also faces a reflection of their educational style. Each segment, each link, each temporal analysis represents a mirror of teaching practice, implicitly showing how the tutor's language influences student understanding. This concept provides valuable teaching in all contexts where communication plays a crucial role. Effectiveness does not depend solely on the content transmitted but also on its ability to be strategically adapted to the available time, specific context, and interlocutor's reactions. Such an approach is particularly valuable in areas like corporate management or negotiations, where success often hinges on the ability to modulate the message based on the moment and circumstances. Choosing the right message at the right time can determine positive outcomes or, conversely, lead to significant failures.
POSR is not just technology but a laboratory for exploring the human dynamics of learning and interaction. The ability to analyze educational conversations in real time could become a model for other areas, such as professional coaching, leadership, and crisis management. In all these contexts, segmenting interactions and analyzing time can provide valuable insights into optimizing decision and action flows. POSR teaches us that every interaction consists of meaningful units, each with its own rhythm and role, and recognizing these units is the first step toward improving any communication-based process.
Finally, there is a broader reflection on the cultural and social impact of tools like POSR. In a world where educational technology is increasingly pervasive, there is a risk of viewing time as merely a mechanical variable to optimize. POSR reminds us that educational time is human time: rich in nuances, vulnerable to attention and emotions, and inseparable from context. This invites not only educators but also corporate leaders and policymakers to consider that innovation is not just about efficiency but about balancing technological precision with human depth. Only in this balance can POSR's true potential be realized—not as a tool to accelerate learning but as a means to make it more meaningful, adaptive, and inclusive.
Source: https://arxiv.org/abs/2411.07598
Comments