“Chimera: Accurate retrosynthesis prediction by ensembling models with diverse inductive biases” is the title of the research conducted by Krzysztof Maziarz, Guoqing Liu, and Hubert Misztela, in collaboration with Microsoft Research AI for Science, Novartis Biomedical Research, and Jagiellonian University. The study addresses the issue of chemical retrosynthesis, that is, predicting the reaction steps required to obtain a target molecule from simple reagents, by leveraging Machine Learning models combined into a single framework. This research fits into the domain of applying artificial intelligence to the synthetic planning of molecules of pharmaceutical and industrial interest.
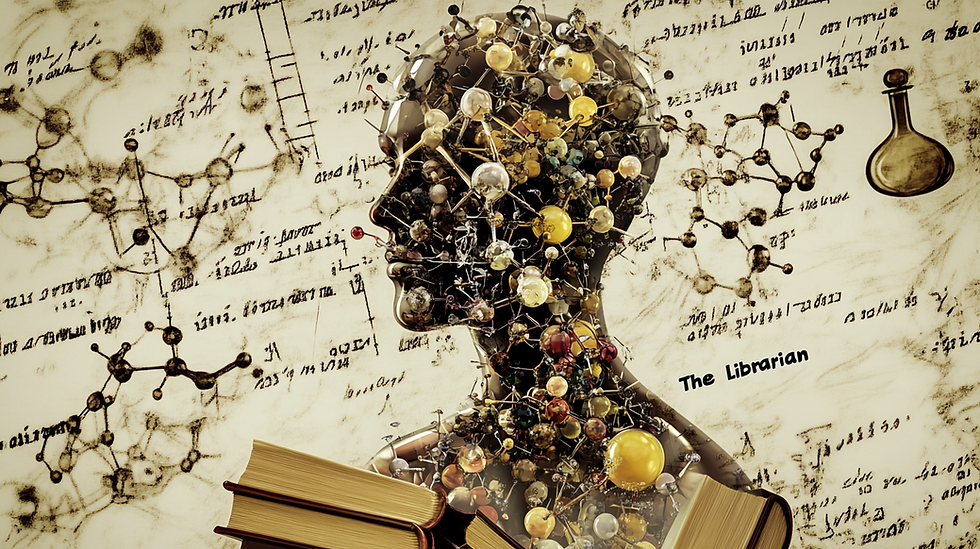
The challenge of retrosynthesis and the role of Machine Learning
Chemical retrosynthesis prediction has long been a critical point in developing new molecules, both in the pharmaceutical field and in related industries. Classical approaches, based on expert systems and manually coded rules, have struggled to scale up to complex or infrequent reactions. The emergence of Machine Learning methods has opened up different perspectives, allowing models to be fed large quantities of known reactions and to extract useful patterns for predicting how to break down a molecule into simpler reagents. In recent years, various models have attempted to capture the logic of retrosynthesis. Some focus on applying transformation rules to the molecular ring, while others concentrate on generating reagents from scratch, starting from the desired product. However, the presence of rare or underrepresented reactions in training data has often limited accuracy.
For business executives or R&D managers, this translates into the need to allocate internal resources to manually verify the suggested routes, slowing the transition from design to experimentation. A tool capable of predicting transformations more robustly and reliably is therefore required.A concrete example: imagine a company needing to identify a synthetic route for a complex bioactive molecule that is scarcely documented in the literature. A traditional ML model might produce incomplete or chemically implausible routes if the molecule differs too much from known cases. Conversely, a more robust model would be able to propose feasible synthetic paths and reduce the burden on chemists who would otherwise have to laboriously develop alternatives by hand.
The Chimera framework: principles and architecture
The heart of the research is expressed through Chimera, a meta-framework that combines retrosynthesis models with different inductive biases. The idea is simple yet powerful: instead of relying on a single model, Chimera integrates models that adopt distinct strategies. Some approaches are based on editing existing molecular templates (template-based), while others generate reagent structures from scratch (template-free). Bringing these viewpoints together provides a broader horizon, allowing different weights to be assigned to suggestions and valuing those that are consistent across multiple sources.
At the core of Chimera lies a specifically optimized ensembling scheme. The system learns to combine the lists of candidate reactions produced by each model, prioritizing those that appear in top positions and are in agreement among several sources. The algorithm learns the ensemble weights to maximize validation accuracy, ensuring that the final predictions are more robust than the individual contributions.For entrepreneurs seeking stable results, this architecture offers a tangible advantage: it reduces uncertainty regarding which proposals to consider. Think of a scenario where a single model provides unreliable hypotheses. The presence of a second model with different constructive biases can highlight better options. A business leader interested in investing in complex chemical projects will find in Chimera a tool that mitigates the risk of relying on a single computational perspective.
Experimental performance on public datasets (USPTO)
Validating on public data is essential for measuring an approach’s effectiveness. Chimera was tested on widely used datasets in the scientific community, such as USPTO-50K and USPTO-FULL, both popular benchmarks for evaluating retrosynthesis. The quantitative results show a significant gain over individual models. For example, considering top-10 accuracy (the probability that the correct reaction appears among the first 10 proposals), Chimera improves by 1.7% on USPTO-50K and 1.6% on USPTO-FULL compared to the state of the art. Although these might initially seem like modest increments, they become substantial when applied to particularly complex molecules.
Every percentage point gained represents a reduction in the manual labor needed to filter out inappropriate reactions. From an operational perspective, if a laboratory manager aims to find reliable routes for dozens of new molecules, even small differences in accuracy translate into significant savings in time and effort. Having a range of options with high chemical consistency, without having to sift through hundreds of implausible solutions, is strategically valuable for accelerating development projects.
Scalability and generalization: results on Pistachio and industrial data
Chimera is not limited to classic datasets. Tested on Pistachio, a more extensive and curated reaction database, the framework also demonstrated high performance on rare or underrepresented reactions, showing strong generalization capabilities. Thanks to the integration of different types of models, Chimera can operate effectively in less explored contexts while maintaining reliability. A practical example: with just 10 Chimera predictions, one can achieve a quality comparable to what a single reference model obtains with 50 predictions, significantly reducing the manual review of solutions.
Moreover, transferring the model without retraining to an internal set of about 10,000 reactions from a major pharmaceutical company (Novartis) confirms the framework’s solidity even when faced with a distributional shift—situations in which the reactions in the new context differ substantially from the training data. Improving over individual models on industrial data highlights a concrete opportunity for companies: reducing the time required to identify viable synthetic routes and lowering uncertainty in chemical research.
Strategic implications and quality of predictions
One key question concerns the actual quality of the proposed solutions. It is not enough merely to obtain the exact target reaction; it is also necessary that the alternative solutions be chemically plausible. Internal tests with organic chemists have evaluated Chimera’s predictions, showing a preference for its suggestions compared to those of single models. This confirms not only the framework’s ability to recover known reactions but also to suggest reagent-product pathways that make sense from a chemical perspective.
For a manager, such a scenario means having a tool capable of proposing not only the “official” route found in the literature but also alternative synthetic paths of comparable quality, ready to be tested experimentally. For instance, if a classic route proves impractical due to the lack of certain reagents, it is helpful to have chemically coherent alternatives suggested by the model. This robustness reduces the risk of impasse, enables more efficient resource use, and accelerates the iterative cycles of design and validation, increasing the chances of identifying interesting molecules for new industrial projects.
Conclusions
The analysis of the Chimera research results suggests a scenario in which combined retrosynthesis models can overcome the limitations of individual approaches. The observation that merging different inductive biases increases the reliability of predictions, even for rare reactions and in new temporal and structural contexts, opens up prospects for integration with existing tools. Companies accustomed to classical systems could integrate Chimera as a decision-support module to identify non-obvious synthetic routes, reducing waste and minimizing failed laboratory attempts. Compared to existing technologies, Chimera does not diminish the importance of human experts but rather enhances their work. Unlike similar methods, the ensemble approach provides a broader viewpoint: where a single model tends to propose routes similar to those seen in the past, the interaction of different models produces more dynamic solutions.
For business executives, the increased stability offered by multiple models can contribute to more effective research strategies, decreasing uncertainty and optimizing workflows.The future scenario remains open: one could consider extending the Chimera framework to even larger models or integrating additional sources of information, such as internal corporate databases enriched with specialized knowledge. The objective is not to replace chemists but to provide them with more flexible and accurate tools for navigating an increasingly complex molecular landscape. In this sense, Chimera could become an important component in an innovation-oriented strategy aimed at generating new synthetic ideas and maximizing the potential of advanced chemical research.
Source: https://arxiv.org/abs/2412.05269
Comments