Scientific research is increasingly becoming a complex challenge, requiring the ability to synthesize decades of studies. The current human capacity to process information has become inadequate in the face of the enormous amount of publications produced daily. In this scenario, Large Language Models (LLMs), trained on a vast corpus of scientific literature, emerge as a promising solution for integrating and predicting new results, often with greater efficiency than human experts. A recent study, published in the journal Nature Human Behaviour, introduced BrainBench, an innovative benchmark designed to evaluate the ability of LLMs to make predictions in the field of neuroscience, directly comparing them with experts in the field.
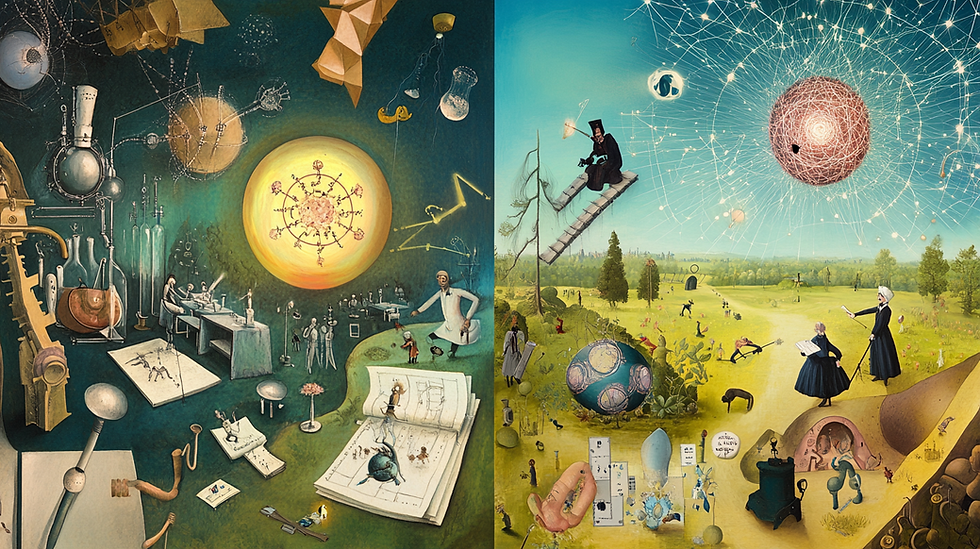
BrainBench and the Prediction Challenge
BrainBench is a benchmark specifically designed to test the ability of language models to predict the outcomes of neuroscience experiments. The structure of BrainBench includes the presentation of modified versions of scientific abstracts, allowing evaluation of the ability of LLMs to distinguish between plausible and altered results. The peculiarity of BrainBench lies in its "forward-looking" nature, meaning its ability to measure the predictive ability of LLMs in new situations, rather than merely verifying their ability to recall known information. This approach differs from other benchmarks that are primarily "backward-looking," such as PubMedQA or MMLU, where questions are about recalling existing knowledge. In BrainBench, two versions of a scientific abstract are presented, one original and one with modified results, and the participant's task is to identify which version is correct.
The benchmark includes case studies from five subcategories of neuroscience: behavioral/cognitive, cellular/molecular, systems/circuits, disease neurobiology, and development/plasticity/repair. This approach ensures a broad and representative coverage of different areas of neuroscience, making the prediction task particularly challenging. It has been observed that language models outperformed human experts in accuracy in all these subcategories. Specifically, the average accuracy of LLMs was 81.4%, while human experts reached only 63.4%. Even limiting the analysis to human experts with the highest self-assessed level of competence, accuracy reached only 66.2%, still lower than that of LLMs.
Another interesting aspect is the evaluation of models of different sizes. For example, smaller models like Llama2-7B and Mistral-7B, with 7 billion parameters, achieved performances comparable to larger models like Falcon-40B and Galactica-120B.
Furthermore, it emerged that models optimized for dialogue or conversational tasks (such as "chat" or "instruct" versions) performed worse than their base counterparts. This suggests that aligning LLMs for natural conversations might hinder their scientific inference abilities.
The accuracy of LLMs was also measured based on their ability to reduce "perplexity," which indicates the level of surprise the model feels towards a text. The models showed a significant improvement when they could access complete contextual information, rather than focusing on local parts of the text. This demonstrates how the ability to integrate information at a global level is one of the keys to their success compared to humans.
Overall, BrainBench represents an innovative method to evaluate not only the ability of LLMs to recall information but also their ability to generalize and predict the outcomes of experiments never seen before. The approach is based on the use of modified scientific abstracts, where the results of studies are substantially altered, to verify whether the models can distinguish between alternative versions of experiments. For example, an original abstract might report that stimulation of a specific brain area increases a certain activity, while the modified version might indicate a decrease in activity. BrainBench evaluates whether the model can determine which of the two outcomes is more plausible, using methodological information and details provided in the abstract.
This method requires that the models not only identify changes in the results, such as an increase or decrease in brain activity, but also relate them to the rest of the information in the abstract, such as the method used or the logic behind the discovery. In this way, BrainBench measures the ability of LLMs to integrate contextual and methodological information to make coherent inferences about new situations, simulating a scientific discovery process.
The goal of this evaluation is crucial to understanding the potential of LLMs in supporting scientific research, especially in complex fields like neuroscience, where coherence between method, data, and results is essential. This approach does not merely test the memorization of information but explores the ability of models to think critically and contribute to the interpretation and generalization of scientific knowledge.
Why Are LLMs So Powerful in Prediction?
A key element of the success of LLMs is their ability to integrate information from multiple sources and handle the complexity of different levels of detail, as evidenced by tests conducted with BrainBench. Specifically, when LLMs were tested using only single sections of the abstracts, their performance dropped drastically. On the other hand, with the integration of the entire abstract content, including methodology, background, and results, their predictive capability increased significantly. This suggests that LLMs can take advantage of the synergy of different pieces of information to formulate more precise predictions.
Moreover, the ability of LLMs to generalize information, even when noisy or potentially redundant, represents a competitive advantage. BrainBench showed that models like BrainGPT, trained on a specific corpus and enriched through techniques such as Low-Rank Adaptation (LoRA), achieved 3% higher performance than standard models. This improvement indicates how targeted customization and training on high-quality data can make LLMs extremely effective tools for predicting scientific results.
The LLMs' approach to prediction relies on architectures such as Transformers, which allow precise modeling of relationships between elements of the text. This approach is particularly useful in neuroscience, where the phenomena to be analyzed often involve complex and interdependent data. Thanks to their billions of parameters, LLMs can identify patterns and correlations that escape human experts, making them suitable not only for predicting experimental results but also for suggesting new research directions.
Another factor explaining the success of LLMs in prediction is their ability to adjust behavior based on confidence signals. LLMs use the difference in perplexity between versions of abstracts to calibrate their confidence in responses, which results in overall greater reliability. This level of calibration was a key factor in surpassing human experts, as it allowed the models to identify correct answers with greater certainty, especially in the more complex cases.
In summary, the ability of LLMs to process enormous amounts of data, integrating information at different levels of detail and effectively handling complexity, makes them powerful tools for prediction in complex scientific fields. Their performance on BrainBench demonstrates that they are not only capable of competing with human experts but also significantly outperforming them, opening up new possibilities for using AI in supporting research and scientific discovery.
BrainGPT: A Model Tailored for Neuroscience
BrainGPT is a large language model, further specialized beyond general LLMs through specific fine-tuning on the neuroscience corpus. This adaptation was achieved through the Low-Rank Adaptation (LoRA) technique, which allowed the addition of over 629 million new weights within the structures of the Mistral-7B model, equivalent to about 8% of the total number of weights of the base model. This approach made it possible to optimize the model for neuroscience tasks, improving the ability to predict experimental results.
The training of BrainGPT involved over 1.3 billion tokens from neuroscience publications collected between 2002 and 2022, covering a total of 100 scientific journals. The data was extracted using the Entrez Programming Utilities (E-utilities) API and the Python package pubget, to ensure a high-quality and relevant dataset. This massive data corpus provided the model with a broad context for understanding and predicting neuroscience outcomes.
LoRA was chosen for its efficiency in adapting pre-trained models. Instead of retraining the entire model, LoRA inserts low-rank adaptation matrices into Transformer blocks, which are then specifically trained to update the model's behavior in a specific domain of knowledge. This process was particularly effective for BrainGPT, leading to about a 3% improvement in performance on BrainBench compared to general models, as evidenced by the conducted tests.
Analysis of the results showed that the LoRA technique not only improved the model's overall accuracy but also reduced the perplexity of correct answers (t(199) = 15.7, P < 0.001, Cohen's d = 0.25), indicating more effective specialization for neuroscience material. This improvement was achieved with relatively limited computational resources: the fine-tuning process required about 65 hours of computation on Nvidia A100 GPUs, using four units in parallel.
An interesting aspect of BrainGPT is its ability to be continuously updated with new neuroscience data. Using complementary approaches such as retrieval-augmented generation (RAG), the model could be constantly aligned with the latest literature, thus ensuring always up-to-date and relevant performance. In this way, BrainGPT can evolve into a tool not only for prediction but also for suggesting and supporting the planning of future experiments.
This lays the foundation for increasingly close collaboration between human researchers and artificial intelligence models, expanding the possibilities for scientific discoveries in a complex field like neuroscience.
The Challenge of Confidence Calibration
Confidence calibration turns out to be a key element in studying the performance of large language models (LLMs). Research has shown that there is a positive correlation between the confidence expressed by the models in their answers and the accuracy of these answers. Specifically, when models were highly confident, their predictions were significantly more accurate. This relationship was quantified using logistic regression, highlighting a significant relationship between perplexity (an indicator of how predictable a model considers a given text) and the correctness of the answers provided.
It was found that language models perform better when they can clearly distinguish between correct and altered versions of a text. This ability was measured using a statistical tool called "Spearman correlation," which indicates how strongly two variables are related. In our case, the value of 0.75 shows a very strong relationship: the better the models were at noticing differences in texts, the more accurate their answers were. The result was confirmed with high certainty, with a very small margin of error (±0.08 in 95 out of 100 trials).
This calibration has a crucial impact on decision support systems, where the model evaluations can integrate with human judgment. For example, by dividing results into twenty confidence bands, it was found that at the highest levels of confidence, the average accuracy exceeded 85%, while at the lowest levels it was around 55%. These results highlight the effectiveness of calibration, as both models and human experts showed the ability to accurately assess their own confidence concerning the probability of success. This capability enables more effective synergy between automatic predictions and human oversight.
Another relevant aspect that emerged from the study concerns the differences between models and humans in perceiving the difficulty of the same tasks. Although the average correlation between difficulties perceived by LLMs and those by human experts was only 0.15, among different models the correlation rose to 0.75. This data indicates a complementarity between the areas where humans and models respectively show strengths or weaknesses. Such characteristics can be leveraged to improve collaboration in decision-making processes.
Finally, it was highlighted how confidence calibration not only increases the accuracy of predictions but also contributes to creating a context of trust in the use of LLMs as support tools for research. The ability of a model to indicate the level of confidence in its answers is an essential aspect for the responsible and effective use of these technologies, especially in the scientific field. This allows scientists to rely on these tools for specific tasks while maintaining critical control over the overall decision-making process.
Future Implications: Human-Machine Collaboration
The success of BrainBench and BrainGPT raises a series of crucial questions about the future of science and the role of LLMs in scientific research. If, on the one hand, these models prove capable of accurately predicting experimental results, it is possible to imagine a future in which LLMs become an integral part of the scientific discovery process. These tools could suggest to researchers which experiments to conduct, identify promising results, and guide data interpretation.
A crucial aspect will be to ensure effective integration between the computational power of LLMs and human ingenuity. LLMs are capable of managing a quantity of scientific data far exceeding human capacity, rapidly processing thousands of articles, and providing connections between studies that often elude experts. However, human intuition, creativity, and the ability to contextualize a specific problem remain irreplaceable to ensure that discoveries have a significant impact and are directed towards useful and innovative applications.
To maximize the potential of human-machine collaboration, it will be necessary to develop support tools that help researchers understand LLM predictions and assess their confidence. For example, user interface-based tools that visualize an LLM's confidence level in a specific prediction could improve transparency and facilitate a more informed use of AI-generated recommendations. In particular, it could be useful to implement visualizations that show the differences in perplexity between correct and altered versions of abstracts, allowing researchers to better understand the basis on which an LLM has made its prediction.
Another interesting implication concerns the possibility of using LLMs to generate innovative experimental hypotheses. The ability of language models to identify hidden patterns in data could lead to the formulation of hypotheses that would otherwise not be considered, thus accelerating the pace of discoveries. However, it is essential that researchers maintain a critical approach, carefully evaluating the predictions and hypotheses generated to avoid the risk of blindly following a direction suggested by AI, without considering the possibility of unexpected or contradictory results.
Moreover, human-machine collaboration could benefit from continuous interaction and mutual adaptation. For example, LLMs like BrainGPT could be trained using explicit feedback from human researchers, continuously improving their ability to provide relevant suggestions. Similarly, human experts could develop new experimental or theoretical methodologies based on the suggestions of LLMs, creating a virtuous cycle of innovation and discovery.
However, one of the main risks is relying too heavily on LLM predictions, especially when these suggest a research path that might seem safer or more promising. This could lead to a reduction in the exploration of less obvious but potentially groundbreaking hypotheses. The risk is that science becomes less exploratory and more oriented towards an optimization logic based on predictive models, which could limit the potential for truly innovative discoveries.
Finally, the complementarity between LLMs and human researchers could be further enhanced by developing specialized models for different fields of knowledge. As demonstrated with BrainGPT, a model trained on a specific corpus improved its performance compared to generalist LLMs. Extending this approach, we could imagine a network of highly specialized LLMs, each with a deep understanding of a specific field, collaborating to solve complex problems, creating a knowledge ecosystem where the analytical capabilities of machines and human creativity enhance each other.
In summary, the future of scientific research could see increasing integration between LLMs and human scientists, with these models becoming not only support tools but genuine partners in discovery. The key to success will be maintaining a balance between reliance on LLM predictions and human creativity and independent thinking, ensuring that innovation remains at the heart of the scientific process.
Conclusions
The ability of language models to surpass human experts in neuroscience raises profound questions about the future of scientific research and the dynamics of human-machine collaboration. This phenomenon is not merely a matter of computational efficiency but opens strategic perspectives on how we address the complexity of knowledge and organize intellectual resources. Through tools like BrainBench and specific models like BrainGPT, LLMs not only demonstrate their ability to compete with human experts but also push us to rethink the value and role of intuition and experience in data-intensive fields.
The superior performance of LLMs is not just a matter of predictive accuracy but reflects a paradigm shift in knowledge management. Their ability to integrate enormous amounts of information, often distributed across different disciplines, redefines the concept of expertise, shifting it from the depth of individual knowledge to the breadth of collective analytical capability. This poses a fundamental challenge to traditional structures of scientific research, where the authority of the expert was a cornerstone. LLMs, with their adaptability and specialization capabilities, could soon become a new standard for validating, predicting, and proposing scientific hypotheses, making the boundaries of expertise more fluid and collaborative.
A crucial aspect is the emergence of a "calculated confidence" that LLMs can offer, redefining the relationship between prediction and decision. The ability to calibrate confidence based on perplexity and communicate it transparently represents a strategic innovation for decision-making processes, not only in neuroscience but also in sectors such as medicine, economics, and engineering. This feature is not merely a technical improvement; it is a model of how humans can learn to manage uncertainties and probabilities in complex situations. Business decision-makers, for example, could adopt this approach to combine quantitative analysis and human judgment, optimizing strategies and reducing risks associated with uncertain decisions.
The risk of an "optimized but not exploratory" science deserves a broader strategic reflection. If, on the one hand, LLMs can direct researchers towards areas of greater probability of success, on the other hand, they might discourage exploration of less obvious or contrary hypotheses. To avoid this danger, it will be essential to balance the analytical power of LLMs with human creative courage. Companies that invest in innovation models capable of integrating these two dimensions will have a competitive advantage in generating radical and not just incremental solutions.
The human-machine complementarity should not be seen as a simple sum of the parts but as a new knowledge ecosystem where interaction produces emerging value. For example, the idea of continuous feedback between human experts and LLMs represents not only an opportunity to improve technological performance but also a way for humans to learn from perspectives that would otherwise remain inaccessible. This is not a technical detail but a guiding principle for building organizations capable of adapting rapidly to changes and anticipating future trends.
Finally, the specialization of LLMs, as in the case of BrainGPT, opens up new scenarios for a "network of specialized artificial intelligences," where highly focused models work together to tackle complex and interdisciplinary problems. This concept of "distributed intelligence" is not limited to science but extends to businesses, governments, and other areas where success depends on the ability to connect dots across seemingly distant systems. The ability to orchestrate this network will be one of the key competencies of the future, redefining not only how we work but also how we think and innovate.
In conclusion, the future of scientific research could see increasing integration between LLMs and human scientists, with these models becoming not only tools of support but true partners in discovery. The key to success will be maintaining a balance between relying on LLM predictions and fostering human creativity and independent thinking, ensuring that innovation remains at the core of the scientific process.
Comments