AI-Driven Materials Innovation: Transforming Research, Product Development, and Workforce Dynamics
- Andrea Viliotti
- 1 gen
- Tempo di lettura: 13 min
In the United States, a growing body of work explores how advanced artificial intelligence, particularly deep learning, can accelerate scientific discovery and reshape the process of material innovation. A notable example is the research paper “Artificial Intelligence, Scientific Discovery, and Product Innovation” by Aidan Toaner-Rodgers, developed at MIT with the collaboration of economists Daron Acemoglu and David Autor. This research scrutinizes how the introduction of a specialized deep learning tool in a large industrial laboratory impacts scientists’ productivity and alters the strategic decisions companies make about which projects to pursue.
The study focuses on a cohort of 1,018 scientists working for a major corporation eager to expedite the creation of novel materials by tapping into AI-driven techniques. In doing so, the paper illustrates how AI can bring significant changes not only to the nuts and bolts of research and development (R&D) but also to the skill sets and roles of the workforce involved. Its findings are crucial for executives and entrepreneurs alike, highlighting both the substantial gains in patent activity and the broader diversification of prototypes. Yet the authors also discover an uneven distribution of these benefits among different categories of researchers—an imbalance that intensifies the need for strategic oversight and training.
A recurring theme is that human expertise remains indispensable for interpreting and validating the AI’s output. The best outcomes surface when experienced scientists leverage the model’s suggestions effectively. This synergy of technology and specialized knowledge underscores the transformative potential of AI-driven materials innovation for corporate management and scientific progress and beyond.
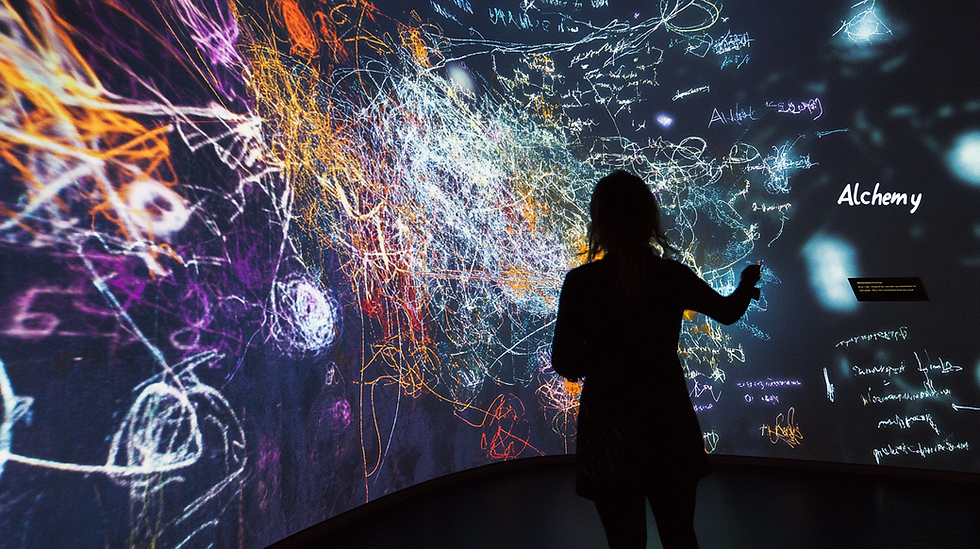
How AI-Driven Materials Innovation Expands Corporate Horizons
The study offers a detailed narrative about how AI-driven materials innovation might spur the development of new materials in critical industries—think consumer electronics, medical devices, or advanced manufacturing. Historically, creating a new type of functional material often involved a significant amount of guesswork. Researchers spent countless hours devising hypothetical combinations of chemical elements, measuring their properties using expensive tools, and discarding a large portion of these attempts as unviable. High failure rates and the need to control costs pushed many R&D teams toward incremental adjustments rather than bold leaps into the unknown.
In contrast, the deep learning system described in Toner-Rodgers’s paper uses graph neural networks (GNNs) to propose fresh “recipes” for new chemical structures. In essence, the algorithm is trained on substantial datasets detailing known materials and their physical or chemical traits—such as mechanical strength, resistance to temperature extremes, or unique optical properties. Building on that foundation, it synthesizes new formulas by scanning the statistical relationships in the data. Additional methods—often described as “diffusion models” and sophisticated probabilistic estimations—help identify solutions that might never have been tried but show promise on paper.
It is vital to note that this AI-driven process is not purely automated. The scientists still take center stage in verifying the feasibility of the AI’s recommendations. They identify which material proposals are suitable for lab synthesis, weeding out those that might fail a practical test. The MIT researchers had a prime vantage point to witness these steps, as the rollout of AI tools occurred in several waves across various teams, allowing for direct comparisons between those already using the AI tool and those who had not yet received access.
This staggered adoption schedule formed the backbone of a robust research design. It enabled the authors to isolate the effect of the AI system by comparing the performance of scientists actively engaging with it to the outcomes of scientists still working under conventional protocols. As a result, the study captured both quantitative metrics—like the number of patents produced, the novelty of the chemical structures, and the eventual prototyping rate—and qualitative shifts in how scientists spend their time and adapt their approach to problem-solving.
Unprecedented Advances in Material Science with AI
Across the board, results point to a marked uptick in productivity following the introduction of the AI platform. Scientists using the system saw a surge in the number of new formulas generated, many of which possessed unique chemical backbones or properties not previously explored in the lab’s portfolio.
This had a ripple effect on multiple R&D metrics:
Higher Count of Newly Validated Materials
The study reports a 44% increase in new materials validated by researchers who had switched over to using the AI tool. These materials tended to align better with the performance targets R&D teams had in mind, suggesting that the AI’s recommendations were not just more numerous but also more precisely tailored.
Growth in Patent Applications
Patent filing activity rose by 39% among the scientists with AI access, an important gauge of how well a company can protect its intellectual property and achieve a competitive edge.
Increased Prototyping
Moving from the realm of theoretical studies to creating tangible prototypes, the study found a 17% jump in the number of lab projects that reached an advanced stage of physical development.
One of the more intriguing findings is that these increases are not limited to volume. The study also notes an uptick in the intrinsic novelty of the discoveries. Patents began referencing technical terms that were new to the corporate lexicon, and entire product lines emerged that would have seemed impractical in earlier eras. This challenges the idea that AI might only recycle existing knowledge or push scientists to remain in familiar territory. Instead, it appears that the algorithm actively explores undercharted sections of the design space, occasionally coming up with materials that deviate significantly from existing norms.
Balancing AI and Human Expertise in Material R&D
Yet, the successful exploration of these uncharted territories hinges critically on the expertise of human scientists. When faced with large volumes of AI-generated suggestions, researchers with considerable knowledge in chemistry, physics of materials, or advanced synthesis techniques can rapidly spot which leads are truly viable. They can also detect “false positives”—seemingly impressive solutions that fail under the practical constraints of manufacturing, cost, or long-term stability.
In the absence of robust human judgment, the AI might generate a flood of marginally useful formulas. Although the cost of creating new ideas with AI drops significantly—for example, reusing computational simulations is much more economical than running each set of experiments from scratch—there is a separate cost in weeding through poor-quality recommendations. The research suggests that scientists began devoting a larger share of time to screening, refinement, and validation, while automatic generation consumed a smaller chunk of the overall workflow. This transformation of research routines has fundamental implications for how labs structure their teams and set budgets for various research phases.
Tackling Challenges in AI-Driven Research Designs
One of the aspects that makes Toner-Rodgers’s analysis especially persuasive is the multi-phase experimental design. The laboratory introduced the AI tool to different groups of scientists in a staggered manner, using something akin to a randomized assignment. Because of that structure, the researchers could observe real-time changes in both the group that had immediate AI access and the group awaiting the rollout.
Interestingly, the comparison groups did not show a drop in motivation or productivity—they did not, for instance, scramble to file as many patent applications as possible for fear of losing out. That observation helps debunk a simplistic assumption that the introduction of AI mainly creates an “arms race” dynamic where everyone is desperate to outdo the competition, whether or not the tool itself has inherent merits.
To bolster their findings, the authors documented each step of the scientists’ work—from scanning academic literature to setting up real-world experiments—and tracked how these activities evolved. A sharp spike in validated materials signaled that, on average, AI suggestions were valuable. However, deeper data show a growing load of “false starts” for some teams, underscoring that AI systems can produce misleading ideas if not properly curated.
Still, for teams capable of navigating those pitfalls, the payoff was substantial. They leveraged the AI tool not merely to add incremental variants to existing formula sets but to conceive entire new categories of compounds with higher odds of commercializing. This progression was especially notable in areas where advanced mechanical or thermal properties are vital, indicating that for certain specialized domains, AI drastically broadens the design range researchers consider plausible.
Addressing Workforce Inequality in AI-Powered Labs
One of the more socially and organizationally significant findings is that the gains from AI are not spread evenly across the workforce. The study draws attention to a phenomenon in which “top scientists”—those who were already highly productive before the AI introduction—reap the greatest improvements, often doubling their output. Meanwhile, scientists in the lower-performing tiers see more modest benefits, if any at all.
Why does this disparity matter? For one, it magnifies existing inequalities. If a laboratory or a company prizes patent generation as a metric of success, then the “star performers” capable of handling AI-driven workflows get even further ahead, both in prestige and in compensation. This dynamic could eventually spur a reorganization of R&D hierarchies, with management teams choosing to invest more resources in the high achievers while phasing out less productive personnel. Indeed, the paper points out that the company studied eventually downsized a small subset of researchers who consistently underperformed, effectively consolidating resources among those most adept at leveraging AI.
From a talent management standpoint, this reality prompts questions about training and recruitment. Could specialized upskilling programs help mid-tier researchers improve their capacity to filter AI proposals effectively, thus narrowing the performance gap? Or will the workforce naturally gravitate toward intensifying the dominance of the most knowledgeable experts?
Interviews with the scientists revealed that domain knowledge—like familiarity with prior attempts, specific interactions among chemical elements, and unique conditions for synthesis—is crucial for sorting the good leads from the worthless ones. The massive influx of computationally suggested formulas does not negate the need for seasoned judgment. On the contrary, it heightens the need for people who can quickly detect whether an algorithmic output is truly workable. These dynamic challenges the perception that “AI can replace experience” and instead places a premium on those who can blend sophisticated computational tools with a deep grasp of the field’s underlying principles.
Strategic Shifts in Management with AI in R&D
Business leaders face a dual set of promises and perils when contemplating how best to incorporate AI into R&D. On one hand, the data highlight significant gains in the speed and volume of patentable ideas and prototypes. The corporation studied saw new product lines emerging that would have been unlikely under traditional practices. Such leaps are especially enticing in high-stakes industries where being first to secure a patent can lead to market dominance and robust revenue streams.
On the other hand, these breakthroughs require an equally robust infrastructure for testing and vetting. AI can generate a near-endless supply of theoretical solutions, but a company must also invest heavily in the lab capacity, engineering teams, and pilot production lines needed to turn those theories into tangible products. If such downstream resources are in short supply, the organization might experience bottlenecks, leading to frustration and diminishing the real value of AI-driven innovation.
Additionally, the study suggests that once AI starts delivering promising leads, management must address tension between building upon successful existing products and venturing into uncharted territory. While incremental improvements remain essential for stable revenue, the AI tool fosters exploration of dramatically different approaches that might demand substantial new investments and greater lead time to get to market. Deciding how to allocate resources between these parallel R&D channels becomes a critical strategic question.
Licensing and data ownership concerns also come to the forefront. As more businesses rely on proprietary machine learning solutions or external cloud-based platforms, safeguarding intellectual property becomes complicated. The underlying code, training datasets, and resulting materials constitute valuable assets that may require specialized legal arrangements. Large corporations may opt to develop in-house AI solutions, thus keeping full control of the knowledge base. Smaller firms could partner with vendors or academic institutions, potentially sacrificing some independence but acquiring immediate access to advanced tools that would be impractical to build from scratch.
Overall, Toner-Rodgers and his collaborators posit that AI might accelerate innovation in ways that macroeconomic literature has historically underemphasized. While AI’s role in assembly lines and supply chain optimization is well established, its contribution to frontline scientific discovery could exert an even more profound impact, especially if companies learn to integrate these tools effectively into their R&D pipelines. Yet the authors caution that any robust adoption plan must factor in labor dynamics, intellectual property management, and the strategic realignment of R&D resources.
Adapting Workforce Morale in the Age of AI
A lesser known yet critical dimension of this AI adoption story is the effect on job satisfaction. The study’s surveys reveal that the overall satisfaction levels among researchers dropped by 82% (as measured by an internal metric) due mainly to diminished feelings of creativity. Many scientists expressed the sentiment that their primary enjoyment stemmed from the imaginative act of conceiving new compounds. In this new environment, the machine handles most of the “ideation” phase, and human participants are left predominantly with the job of evaluating and filtering.
While such a shift in responsibilities yields tangible gains in productivity and patent counts, it can erode the sense of personal fulfillment many researchers derive from the creative aspects of their job. Even high-performing scientists, who see their overall productivity rise, sometimes report a hollow victory. Their success feels less tied to their individual ingenuity and more to the fact that they have become efficient gatekeepers of the AI’s output.
Consequently, management teams must walk a fine line. As AI becomes more ingrained in R&D, the workforce might split into those who thrive under this new mode of work—often the experts with deep knowledge and strong screening abilities—and those who feel disenchanted because they are no longer applying the same level of intellectual originality. Over the long term, that disenchantment could undermine an organization’s culture of innovation, particularly if it triggers the departure of employees who once brought vitality to the team.
On a more optimistic note, the data show that the majority of respondents do understand the capacity of AI to accelerate the pace of scientific exploration, and many express a willingness to “reskill” or further refine their expertise to stay aligned with changing job demands. Some see a silver lining: freed from manual data searches, they can focus on analyzing the deeper rationale behind which ideas get flagged by the AI, creating a knowledge feedback loop that enriches both the human expert and the machine-learning system.
Expanding AI’s Impact Beyond Material Science
Although Toner-Rodgers’s case study centers on materials science, the authors propose that the same pattern will likely play out in any field where researchers must sift through countless possible configurations—pharmaceuticals (e.g., discovering new drug molecules), advanced robotics (e.g., designing novel sensor-actuator assemblies), climatology (e.g., modeling global climate variables), or even mathematical conjectures in pure research. Any problem domain with a vast “solution space” can benefit from AI’s capacity to propose creative possibilities grounded in data patterns, but such a domain also depends on human discernment to validate those possibilities in real-world contexts.
From a managerial standpoint, the biggest lure is the promise of drastically reduced time-to-market for breakthroughs. Managers at large corporations see AI solutions as a way to compress multi-year R&D cycles into significantly shorter spans, thus beating competitors or securing an early patent in a niche area. This impetus for faster innovation might reshape how companies’ budget for R&D, how they organize interdisciplinary teams, and how they distribute risk across a portfolio of short-term and long-term projects.
However, the authors repeatedly emphasize that greater speed in generating ideas does not magically ensure those ideas will be grounded in practicality or cost-effectiveness. The presence of AI makes it easier to produce large volumes of speculation, and some portion of that speculation will always be illusory unless curated by skilled personnel. This inherent dependence on expert oversight underscores the continuing need for robust education, specialized hiring, and dynamic upskilling programs. The study even cites the reduction of about 3% of the staff in the company’s less experienced ranks, a move that the firm believed was necessary to optimize the synergy between advanced modeling and seasoned human assessment.
The Future of AI in Sustainable Scientific Innovation
Another intriguing takeaway is how AI-driven R&D can prompt the creation of product lines that diverge significantly from a firm’s legacy offerings, thereby expanding market possibilities. Yet these radical new directions require a broader supply chain, additional training for engineers, and possibly new manufacturing processes. Even if the AI tool offers an impressive initial concept, the path to large-scale commercialization can still take years—especially if the technology is fundamentally different from anything the company has produced before.
On a larger scale, there is the question of who ultimately benefits from this accelerated innovation cycle. If, for instance, a handful of multinational corporations perfect AI-empowered labs, they may secure a near-monopoly on cutting-edge patents, potentially raising barriers to entry for smaller competitors. Some might argue that a more democratized model, in which open-source AI tools help smaller labs, fosters healthy competition and innovation. But that, too, requires accessible training resources, robust data-sharing channels, and frameworks to protect intellectual property without stifling creativity.
Toner-Rodgers’s study projects that as AI models become more sophisticated, we are likely to see an even greater impact on how scientific research unfolds in industrial settings. Costs associated with experimentation may continue to fall, encouraging a surge in bold product proposals. The flip side is that researchers might feel less ownership of projects where AI has done much of the conceptual heavy lifting, potentially fueling the ongoing talent reshuffling and motivation dilemmas mentioned earlier.
In the face of these rapid advances, it remains critical for R&D directors and senior management to nurture a balanced workplace. The best outcomes will likely come from frameworks that keep top scientists motivated to push boundaries while ensuring mid-level talent receives sufficient training and involvement in truly creative tasks. This environment can help maintain a sense of purpose and collective problem-solving, which, over time, can strengthen a company’s capability to integrate computational insights with human-driven expertise.
Conclusions
Toner-Rodgers’s research reveals a vivid picture of how advanced deep learning can dramatically enhance an organization’s capacity to devise and patent novel materials, offering tantalizing prospects for corporate growth. The empirical data and interviews paint a scenario in which AI is not merely a tool for incremental improvement but a mechanism that accelerates the very formation of new scientific ideas. By analyzing nearly every step of the R&D process and examining how teams responded to the technology, the study offers a nuanced view that goes well beyond standard assessments of productivity gains.
Nonetheless, the findings underscore a more complex landscape than one might expect. While overall productivity and patent numbers rise, there is also a stark divergence in performance across different skill levels, which in turn influences hiring practices, training programs, and corporate structures. Beyond pure metrics of efficiency, the study highlights significant shifts in job satisfaction and creativity, along with the need for advanced screening competencies—a blend of algorithmic insight and deep, experience-based knowledge.
Ultimately, these observations inform corporate leaders that adopting AI tools for materials research (and likely for other scientific domains) is not just a matter of plugging in a new software platform. It demands a serious reevaluation of internal roles, the creation or enhancement of high-level “judgment” positions, a willingness to invest in and empower top experts, and thoughtful planning for the workers who might feel their creative drive diminished. The journey toward AI-augmented science involves recalibrating an entire organizational ecosystem to tap into the promise of large-scale automation without losing sight of the intangible spark that fuels human ingenuity.
As companies look to the future, they will find themselves balancing the incredible promise of more efficient and wide-ranging innovation with the challenges of fostering an engaged and skilled research community. Those that succeed in melding computational power with domain-specific expertise stand poised to achieve dynamic leaps in product development. Yet they must remain attentive to the people behind the process—ensuring that passion, creativity, and collective knowledge continue to propel discovery forward on both scientific and human terms.
Source: https://arxiv.org/abs/2412.17866
Commentaires